Insights
How to adopt a ‘shift-left’ approach to Data and AI Governance amongst employees
- Date 02 Aug 2024
- Filed under Insights
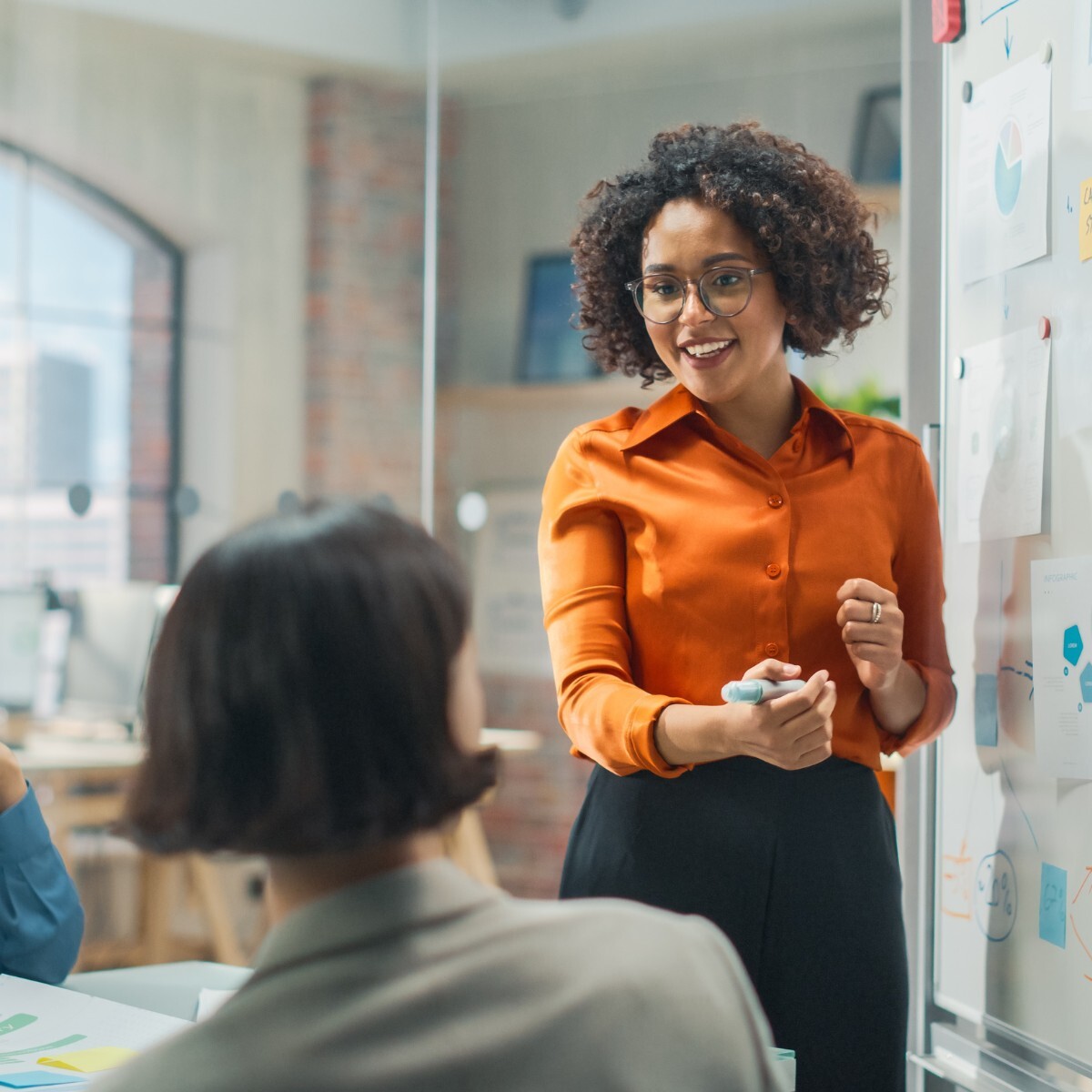
In the age of AI, the latest State of Data report by ITNews says business leaders now face a bewildering array of choices regarding data. From where they store their data (on premises or cloud, in a single location or many) through to how it is analysed and secured.
As leaders realise the true value of their data is defined not only by what it contains, but by how it is managed and used, they are considering the liability that data can present when found in the hands of the wrong people, and the strategies needed to prevent that from happening. It is these choices and strategies that are addressed by Data and AI Governance. The latter of which, Gartner says will soon become just as critical as cyber security.
Data governance encompasses the policies, processes and frameworks that organisations employ to manage and utilise data effectively. However, in many cases, the traditional data governance process has lagged behind the rapid evolution of data management and engineering practices. This disconnect has resulted in a reactive approach to governance, where policies are often implemented as a remedial measure in response to data breaches, compliance violations or issues with data quality.
Data Governance in the data lifecycle
As an IT News State of Data sponsor, NRI was invited to join a Data Governance panel at its recent Data Forum in Sydney.
Elena Green, NRI’s General Manager of Capability Consulting was our panelist, who jointly discussed the emerging ‘shift-left’ approach to Data Governance.
A shift-left approach is where Data Governance considerations occur much earlier in the data lifecycle, promoting a proactive approach. Drawing inspiration from the concept of ‘shifting-left’ in software development, this approach advocates for embedding governance principles and policies into the data engineering process from the outset.
Elena described a fundamental need for this type of strategy in large transformations, such as ERP projects, and especially in the era of AI.
“Firstly, when you look at projects like ‘Lift and Shifts’ which require data migration, they are often prone to failure due to inadequate data management which includes data governance, data quality, data security, data warehousing & BIA, data architecture, and a few other sub-components.
Of the 38% of transformation projects that fail. The biggest reason is always due to data migration. And if you look into those data migration failures, 78% of them failed because of data governance, or lack thereof,” said Elena.
Adding “Modern Data Management tools are fantastic and are mature enough to profile and cleanse data, but if we don’t have the governance in place to say ‘who makes the decisions’, ‘what are the rules’, ‘what are the policies’, it gets stuck. Time gets wasted. And it leads to the collapse of programs.
How can you apply a shift-left approach to governance in the era of AI?
According to Microsoft’s 2024 Work Trend Index Annual Report, 75% of workers are already using AI whether their organisation has issued it or not. This is a big problem for governance teams and one which is almost impossible to control.
But organisations can reduce risks by uplifting the data literacy of employees.
“Educating the workforce is paramount so that they can self-govern. At NRI, what we have put together is an Assessment of your AI outputs against the newly coined ISO standards for AI Management – (ISO/IEC 42001),” said Elena.
The standard provides guidance for organisations to address AI challenges such as ethics, transparency and continuous learning, and take you step by step through the educational path that you need to take.
Describing NRI’s approach, Elena said “We suggest starting with a job centric approach where you view the role that employees are in, the decisions they make, the information they consume when making those decisions, and what data is requested of them.
Then within that journey, what is their use of AI? What are the questions that will need to be asked to interrogate AI tools, models and systems, in order to get effective outcomes?”
Following that, you can set up data literacy programs for the purpose of greater awareness and education, which “empower employees to implement their own governance and assume accountability for their decisions—such as refraining from inputting sensitive information into AI platforms like ChatGPT—organisations can foster a culture of responsible data management”.
Elena also highlights the importance of training personnel to identify AI-generated inaccuracies, known as ‘AI Hallucinations’, and ethical biases.
“It’s essential to view AI outputs as augmented intelligence, a tool to enhance human decision-making rather than replace it.
Adopting these practices will not only elevate data literacy but also enable organisations to harness the anticipated efficiency and effectiveness from AI technologies.”
Download our latest guide ‘Make Microsoft Copilot work for you’.
Working on your AI strategy right now? We’ve documented some the key mistakes organisations are making when it comes to rolling out Copilot, and the practical steps leaders can take to turn experimentation into tangible business impact.